As the release of the United States’ fourth-quarter GDP figures draws near, it has become increasingly evident that forecasting the rate of change for this metric remains a significant challenge. Current projections even within the Federal Reserve’s own models show considerable divergence. For instance, the Atlanta Fed’s GDPNow model currently predicts growth at 3.3%, whereas the New York Fed’s equivalent model estimates a much lower rate of 1.8%.
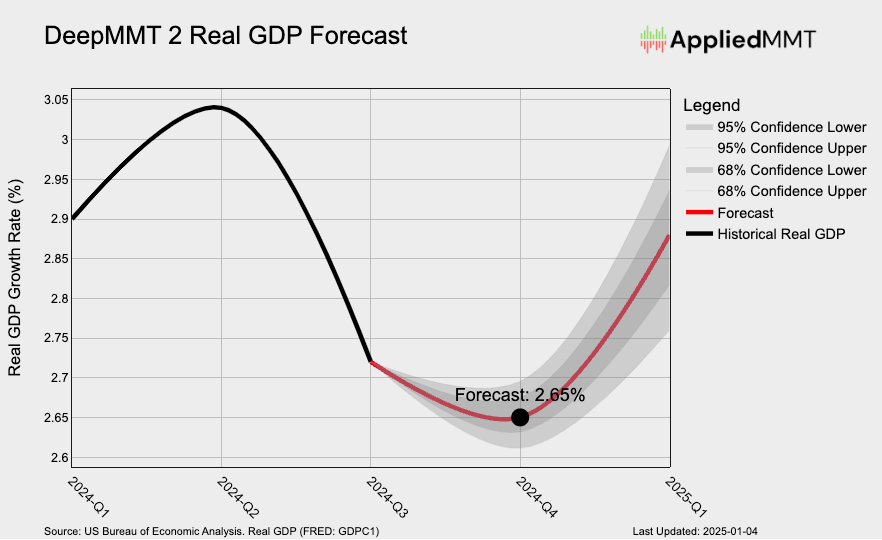
In contrast, the DeepMMT 2 model forecasts GDP growth at 2.65%. While we hold a high degree of confidence in this prediction, it is important to note that even a single day can significantly influence the final reported figure. Unlike traditional forecasting models, which rely on discrete time intervals such as month-to-month or quarter-to-quarter calculations, the DeepMMT 2 model operates in continuous time. Its unique architecture updates each variable every 14 hours, providing a more fluid and dynamic approach to forecasting.
However, the model has exhibited some endogenous behaviors that present forecasting challenges. For example, as of the final day of 2024, the DeepMMT 2 model projects year-on-year GDP growth of 2.65%. Yet, strikingly, just one day later, the forecast spikes to 3.1%. This abrupt shift aligns more closely with the Atlanta Fed’s 3.3% forecast, raising an important methodological question: should we intervene to adjust the model to align with our expectations, or should we allow it to operate as designed and generate its natural outputs?
We have chosen to let the DeepMMT 2 model run without interference. As a system dynamics model, DeepMMT 2's fundamental purpose is to reveal emergent properties without introducing artificial exogenous shocks that lack real-world justification. This commitment to preserving the model’s integrity allows us to better understand the complex and nonlinear dynamics of the macroeconomy rather than forcing the model to fit preconceived notions or externally imposed targets.
In essence, the DeepMMT 2's model functions much like a weather forecasting system. Its nonlinear structure enables it to outperform other models over the long term while maintaining high levels of predictive accuracy in the short term. As the statistician George Box famously remarked, “All models are wrong, but some are useful.”
Our goal is for the DeepMMT 2 model to be both useful and insightful, even if it occasionally diverges from the exact final figure. We are committed to resisting any temptation to compromise its integrity merely to improve short-term alignment, as doing so would undermine its value as a tool for understanding emergent economic phenomena.